MSA Repository "MSAR"
MSAR University's Digital Repository is a documentation and digitization of all university outcomes that are of effective value in the scientific and academic community and reflects the university's image, work, and effective contribution to society Through MSAR Digital Repository, the university managed to collect, store, archive and publish digital content - including documents, audio files, images and data sets - all in a safe place. MSAR is one of the strongest University Digital Repositories in Egypt and documented in the DSPACE community with its latest versions.
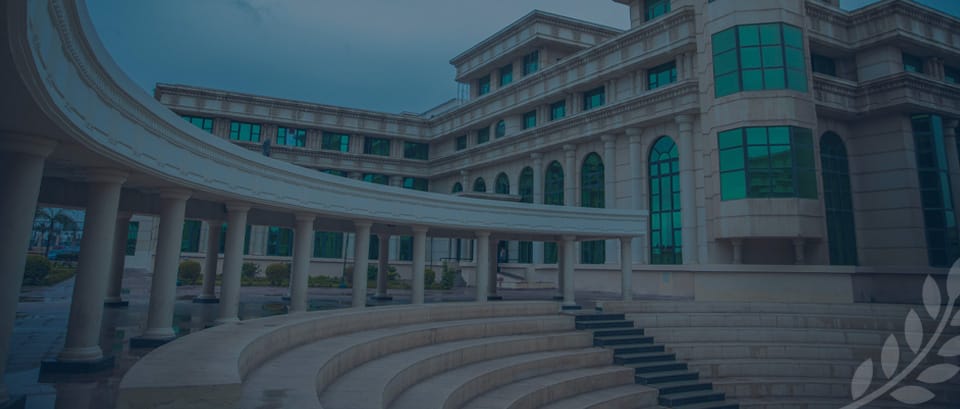
Communities in DSpace
Select a community to browse its collections.
- A Full content for MSA university Faculties Journals
- A Full content for msa university Distinguished Graduation Projects Yearbook
- Images for MSA University " sites - building - landscape "
Recent Submissions
EXT1 as an Independent Prognostic Biomarker in Breast Cancer: Its Correlation with Immune Infiltration and Clinicopathological Parameters
(Multidisciplinary Digital Publishing Institute (MDPI), 2025-03-13) Amira Hossny; Hatem A. F. M. Hassan; Sherif Ashraf Fahmy; Hazem Abdelazim; Mahmoud Mohamed Kamel; Ahmed H. Osman; Sherif Abdelaziz Ibrahim
Exostosin 1 (EXT1) encodes a type II transmembrane glycosyltransferase residing
in the endoplasmic reticulum and plays an essential role in the elongation of heparan sulfate
chain biosynthesis. Additionally, EXT1 may act as an oncogene that could promote cell
proliferation as well as cancer cell metastasis. Herein, we investigated EXT1’s expression
pattern and prognostic value in breast cancer, along with its immunological implications.
Immunohistochemical staining of EXT1 was assessed in 85 breast cancer patients. Patients
were categorized into molecular subtypes, namely luminal A, luminal B, and human
epidermal growth factor receptor 2 (HER2), along with triple-negative breast cancer (TNBC).
Correlations of EXT1 immunostaining with clinicopathological parameters were evaluated.
Furthermore, the correlations of EXT1 expression with tumor immune infiltration and
immune cell surface markers were assessed using TIMER. Moreover, survival analysis was
conducted to reveal EXT1’s prognostic value. EXT1 expression was markedly associated
with the status of the estrogen receptor (ER), molecular subtypes, and recurrence status.
In addition, high levels of EXT1 expression were associated with worse overall survival
(OS) and relapse-free survival (RFS). Analysis of immune infiltration indicated that EXT1
expression was positively correlated with dendritic cells (DCs), macrophages, neutrophils,
CD4+ T cells, and CD8+ T cells, although it showed a negative correlation with the tumor
purity. Overall, this study suggests that the elevated EXT1 expression, particularly in TNBC,
has a positive correlation with poor prognosis and with immune-infiltrated cells in breast
cancer. Therefore, it may emerge as an independent prognostic biomarker, immunological
marker, and potential future therapeutic target for the most aggressive TNBC subtype.
Impact of Temperature Variations on the Viability and Infectivity of Sporulated Eimeria papillata Oocysts in C57BL/6 Mice
(Springer New York, 2025-03-12) Mohammed Albeshr; Rewaida Abdel‑Gaber; Nagham Dkhil; Manal F. Elkhadragy; Esam M. Al‑Shaebi; Saleh Al‑Quraishy
Eimeria is a protozoan apicomplexan parasite that afects animals and poultries. This study investigated how temperature
variations impact the inactivation of sporulated E. papillata oocysts during experimental infections in mice. A total of 20
male C57BL/6 mice were divided into four groups, with fve mice in each group, as follows: Group 1: Control group – Group
2: Infected group inoculated with fresh oocysts (subjected to 23 ℃ for 7 days) – Group 3: Infected group inoculated with
thermal oocysts (subjected to heating conditions at 100 ℃ for 60 min) – Group 4: Infected group inoculated with frozen
oocysts (subjected to freezing at −20 ℃ for 7 days). An oral infection was initiated using 103
sporulated E. papillata oocysts
to inoculate groups 2–4. Biological indicators, including body weight (BW) and feed intake (FI), were evaluated for each
group. Oocyst output was assessed on day 5 post-infection. After sacrifcing the mice, jejunal tissues were collected and
processed for histopathological and biochemical analyses. Our fndings indicated signifcant morphological alterations of
sporulated Eimeria oocysts (i.e. a reduction in oocyst size and rounded shape of sporozoite) after being subjected to heating and freezing conditions. During the in vivo study, temperature treatment data shows signifcant inactivation of oocysts,
with no fecal shedding. This result refects enhancements in biological indicators and histopathological injuries, with the
absence of intracellular Eimeria stages in the jejunal tissues, alongside a restoration of nutrient composition, particularly
carbohydrates and proteins. Signifcant observations were also recorded regarding catalase (CAT), glutathione (GSH), nitric
oxide (NO), and hydrogen peroxide (H2O2) levels. Temperature changes impact the viability of Eimeria oocysts and their
role in coccidiosis. More research is needed on other biotic and abiotic factors in managing the disease.
Eco-Friendly Extraction of Sustainable and Valorized Value-Added Products From Ulva fasciata Macroalgae: A Holistic Technoeconomic Analysis
(Hindawi Publishing Corporation, 2025-02-26) Nour Sh. El-Gendy; M. Shaaban Sadek; Hussein N. Nassar; Ahmad Mustafa
Te present work conducts a detailed technoeconomic analysis of an environmentally friendly zero-waste biorefnery process to
valorize marine Ulva fasciata macroalgae into diferent sustainable value-added products. Te proposed sequential fully integrated
process yielded 34.89% mineral-rich water extract (MRWE), 2.61 ± 0.5% chlorophyll, 0.41 ± 0.05% carotenoids, 12.55 ± 1.6%
starch, 3.27 ± 0.7% lipids, 22.24 ± 1.8% ulvan, 13.37 ± 1.5% proteins, and 10.66 ± 0.9% cellulose. Te Aspen Plus software, utilizing
the nonrandom two-liquid (NRTL) model, was applied for process design, simulation, and technoeconomic analysis. Key fndings
include a positive net present value (NPV) of $49,755,544.90, a high return on investment (ROI) of 485%, and an internal rate of
return (IRR) of 17%. Te anticipated payback period is 7 years, indicating a quick recovery of the initial investment. Tese fndings
confrm that Ulva fasciata is a promising resource in the biorefnery industry, providing a viable and eco-friendly alternative for
the production of bio-based products and a new market for seaweed-based products.
Non-coding RNAs: emerging biomarkers and therapeutic targets in cancer and inflammatory diseases
(Frontiers Media SA, 2025-03-10) Basma Hossam Abdelmonem; Lereen T. Kamal; Lilian Waheed Wardy; Manon Ragheb; Mireille M. Hanna; Mohamed Elsharkawy; Anwar Abdelnaser
Non-coding RNAs (ncRNAs) have a significant role in gene regulation, especially in
cancer and inflammatory diseases. ncRNAs, such as microRNA, long non-coding
RNAs, and circular RNAs, alter the transcriptional, post-transcriptional, and
epigenetic gene expression levels. These molecules act as biomarkers and
possible therapeutic targets because aberrant ncRNA expression has been directly
connected to tumor progression, metastasis, and response to therapy in cancer
research. ncRNAs’ interactions with multiple cellular pathways, including MAPK, Wnt,
and PI3K/AKT/mTOR, impact cellular processes like proliferation, apoptosis, and
immune responses. The potential of RNA-based therapeutics, such as antimicroRNA and microRNA mimics, to restore normal gene expression is being
actively studied. Additionally, the tissue-specific expression patterns of ncRNAs
offer unique opportunities for targeted therapy. Specificity, stability, and immune
responses are obstacles to the therapeutic use of ncRNAs; however, novel strategies,
such as modified oligonucleotides and targeted delivery systems, are being
developed. ncRNA profiling may result in more individualized and successful
treatments as precision medicine advances, improving patient outcomes and
creating early diagnosis and monitoring opportunities. The current review aims to
investigate the roles of ncRNAs as potential biomarkers and therapeutic targets in
cancer and inflammatory diseases, focusing on their mechanisms in gene regulation
and their implications for non-invasive diagnostics and targeted therapies. A
comprehensive literature review was conducted using PubMed and Google
Scholar, focusing on research published between 2014 and 2025. Studies were
selected based on rigorous inclusion criteria, including peer-reviewed status and
relevance to ncRNA roles in cancer and inflammatory diseases. Non-English, nonpeer-reviewed, and inconclusive studies were excluded. This approach ensures that
the findings presented are based on high-quality and relevant sources
Plant-derived Synthesis of Iron Oxide Nanoparticles for Magnetic Hyperthermia and Magnetic Resonance Imaging Applications
(Tsinghua University Press, 2025-03-12) Mohamed Abdelmonem; Romesa Soomro; Norazalina Saad; Mohamed Ahmed Ibrahim; Kim Wei Chan; Emmellie Laura Albert; Emma Ziezie Tarmizie; Che Azurahanim Che Abdullah
The biomedical applications of iron oxide nanoparticles (IONPs) synthesized using environmentally
friendly processes are extremely promising. Using eco-friendly and nontoxic methods is a safer
alternative to conventional chemical synthesis, which generates toxic byproducts. It allows for greater
control over particle size and morphology. The resulting unique magnetic and optical properties of
IONPs enable their use in biomedical applications such as magnetic hyperthermia (MH) and magnetic
resonance imaging (MRI). This review aimed to summarize recent advances in the synthesis,
characterization, and biosafety of IONPs for use in MH and MRI. It also aimed to highlight the
significance of eco-friendly synthesis techniques for producing IONPs with the desired magnetic and
physicochemical properties. Overall, this review elucidated the most efficient methods for utilizing
iron oxide while considering biocompatibility.